Example applications of vision inspection systems
- Automotive Industry: in the automotive sector, vision-based systems are used to inspect components such as engine parts, brake systems, and body panels. These systems utilize high-resolution cameras and image processing algorithms to detect defects like cracks, deformations, and surface irregularities that could compromise vehicle safety and performance.
- Electronics Manufacturing: Automated Optical Inspection (AOI) systems are widely used in the electronics industry to inspect printed circuit boards (PCBs). These systems capture high-resolution images of PCBs and use pattern recognition algorithms to identify defects such as missing components, soldering issues, and misalignments, ensuring that only functional electronics are shipped to customers.
- Textile Industry: Vision inspection systems in the textile industry scan fabrics to identify defects such as holes, stains, and color inconsistencies. These systems use cameras and image processing software to continuously monitor the fabric during production, ensuring high-quality textiles are produced without manual inspection.
- Food and Beverage Production: In the food and beverage industry, vision systems are used to inspect products for contamination and packaging defects. For example, x-ray and infrared imaging technologies can detect foreign objects in packaged foods, while high-speed cameras ensure that labels and seals are correctly applied.
- Pharmaceuticals:Vision inspection systems in the pharmaceutical industry ensure that tablets, capsules, and vials are free from defects. These systems use cameras and specialized lighting to inspect for cracks, chips, and discoloration, ensuring that only safe and effective medications reach consumers.
These examples illustrate the significant role of vision inspection systems in enhancing product quality and safety across various manufacturing sectors. By leveraging advanced imaging and machine learning technologies, these systems provide manufacturers with reliable and efficient tools to maintain high standards and improve operational efficiency.
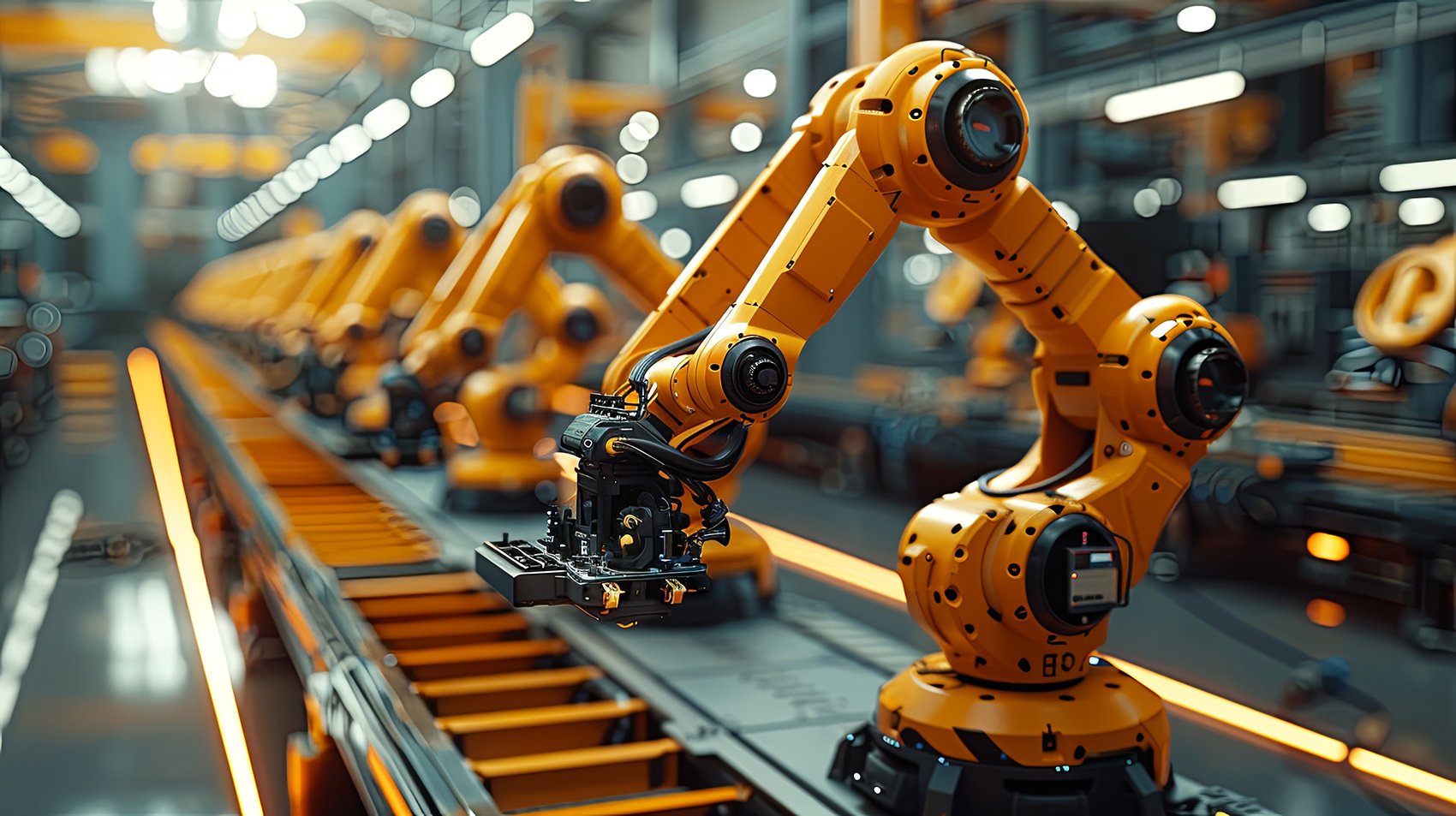
Deep learning has changed quality control for the better
Deep learning has revolutionized defect inspection and quality control in manufacturing by providing unprecedented accuracy, speed, and adaptability. Traditional approaches that use hand-crafted algorithms on vision systems for manufacturing, while effective, have limitations in their ability to learn and adapt to new types of product defects and variations. Deep learning overcomes these limitations by leveraging vast amounts of data to train neural networks that can identify and classify defects with high precision.
Deep learning models, particularly Convolutional Neural Networks (CNNs), have significantly improved the accuracy of defect detection. These models can automatically learn complex features from images, enabling them to detect even the smallest and most subtle defects. For instance, deep learning models can identify micro-cracks in semiconductor wafers, which are crucial for the electronics industry where even minor defects can lead to significant product failures. Thus, deep learning can significantly improve automated inspection.
But the improvements of machine learning in quality control come with some challenges.
Challenges and Opportunities of Machine learning in Quality Control
1. Choosing the right AI-based algorithms for a given problem
The diversity of AI-based algorithms available for automated inspection pose a significant challenge for manufacturers. Different algorithms, based on classification, localization, and segmentation, apply to different problems. Choosing the right algorithm requires understanding the specific requirements of the defect recognition task at hand, which varies significantly across different manufacturing sectors.
- Classification algorithms allow identifying defects within a set of predefined classes (like classifying defective and non-defective microchips in the electronic industry) with CNN models such as ResNet which automatically learn and extract hierarchical features from images recognizing complex patterns
- Localization algorithms allow detecting the presence of defects within the image (like locating surface cracks on automotive parts). Models from the YOLO family can detect and localize multiple defects in real-time, making them suitable for high-speed manufacturing lines
- Segmentation algorithms precisely delineate the boundaries of defects within an image (for example segmenting defects on textile surfaces to identify the exact areas of flaws). U-Net is a popular CNN architecture for segmentation tasks. It excels whenever detailed localization of features within images is required thanks to its encoder-decoder structure that allows it to capture fine details and provide pixel-level segmentation.
- Anomaly detection identifies deviations from the norm without predefined defect classes (for example detecting unusual wear patterns on machinery parts that indicate potential failures). Variational Autoencoders (VAEs) are an example of an architecture used for anomaly detection. These models learn the normal distribution of the data and can identify anomalies as deviations from this distribution. VAEs, in particular, are used to model the normal appearance of components and flag deviations as defects.
How Axelera AI addresses this challenge
At Axelera AI we know that our customers require a wide variety of models for their vision inspection systems. We address this challenge by offering a flexible and performant architecture designed to integrate and adapt to a wide variety of deep learning models at high resolution and high frame rates.
Our unique In-Memory architecture is built with adaptability in mind, allowing seamless integration of new and emerging backbones (the part of neural networks that extract features from the input data, like ResNet and MobileNet). This helps us provide a comprehensive and ever-growing toolkit for defect inspection as part of our Model Zoo.
Our Metis AI platform already supports a wide range of state-of-the-art models and features best-in-class performance across many models. This allows deploying real-time vision inspection systems with multiple high resolution, high frame rate cameras.
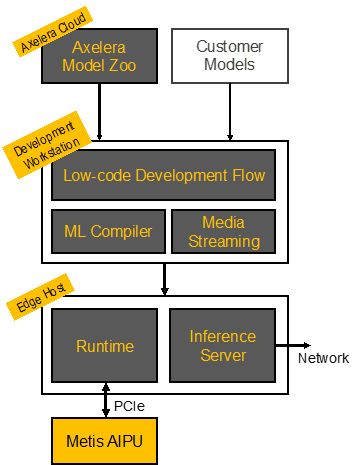
2. Adapting to the uniqueness of each manufacturing line
The fragmentation of problems across different manufacturing lines presents a significant challenge in automated inspection. Each line has unique requirements, materials, and processes, making it difficult to find a one-size-fits-all solution. Consequently, there is a need to adapt models specifically to each problem, often with limited data available for training. Here are the main approaches to solving this challenge:
-
Retraining: involves training a pre-existing model on new data specific to the manufacturing line. This process can be time-consuming and data-intensive but ensures that the model is tailored to the specific defects and characteristics of the production line.
-
Fine-Tuning: is a less resource-intensive method where a pre-trained model is slightly adjusted using a smaller, task-specific dataset. This approach is particularly useful when the available data is limited.
-
Model-Agnostic Meta-Learning (MAML): is a meta-learning technique where a model is trained on a variety of tasks such that it can quickly adapt to new tasks with minimal data. This approach is beneficial in environments where new types of defects may frequently arise.
-
Zero-Shot Learning: allows a model to recognize defects it has never seen before by leveraging knowledge from similar tasks or utilizing descriptive labels. This method is highly advantageous in scenarios with very limited or no defect data.
How Axelera AI addresses this challenge
Axelera AI addresses the challenge of adapting models for machine learning in quality control to the uniqueness of each manufacturing line by providing a robust and flexible solution that minimizes complexity. Our technology does not get in the way of training. Models that have been trained in a hardware-agnostic fashion can be compiled and run on our inference hardware seamlessly and without degradation in accuracy. We provide quantization libraries that automatically handle the optimization of models post-training. This enables efficient deployment without compromising performance and ensures that manufacturers can quickly implement tailored AI solutions to address specific defect inspection needs.
3. Deploying fast and secure inference, anywhere
Deploying AI models that enhance vision systems for manufacturing involves several critical challenges. Axelera AI makes sure they are addressed in order to ensure operational efficiency, privacy, and confidentiality.
- Manufacturing environments often consist of diverse hardware systems from various vendors running different operating systems. A manufacturing line might use a mix of Windows, Linux, and custom real-time operating systems across different machines from vendors like Dell, HP, and Lenovo. Axelera AI’s acceleration platform Metis is available as PCIe or M.2 modules and can be integrated into many hardware solutions, supporting a wide range of operating systems. This ensures compatibility with existing heterogeneous hardware setups.
- Manufacturing systems often have pre-existing software architectures that may be custom-built or rely on widely used libraries like GStreamer. Integrating AI models into these architectures without disrupting existing workflows and processes is achieved thanks to Axelera AI’s Voyager SDK. This SDK provides both low-level APIs and pipelines based on popular frameworks like GStreamer, allowing for easy integration into pre-existing software architectures. This flexibility ensures that AI models can be deployed without significant modifications to existing systems.
- AI inference needs to have low latency and high pixel throughput to meet the demands of real-time defect detection. This is especially important when multiple high-resolution cameras are used, or when the manufacturing process operates at high frames per second (fps). Metis delivers datacenter-grade performance at the edge, ensuring low latency and high throughput necessary for real-time defect detection. This capability is crucial for maintaining operational efficiency in high-speed manufacturing lines with multiple high-resolution cameras.
- Manufacturing data often includes sensitive information that cannot leave the premises due to privacy and confidentiality concerns. Axelera AI’s on-premises AI solution, addresses confidentiality and privacy concerns by ensuring that all data processing occurs within the manufacturing facility.
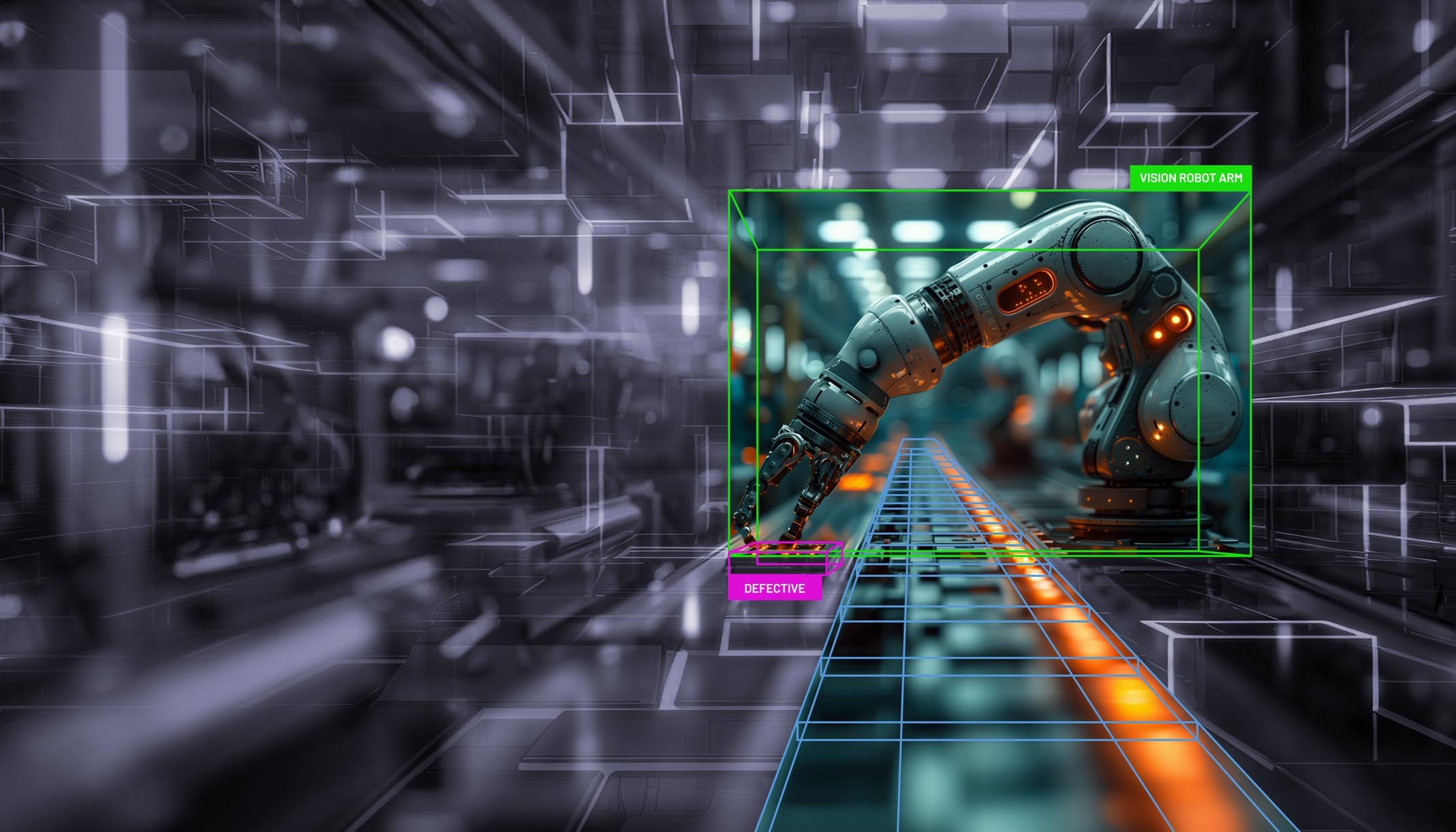
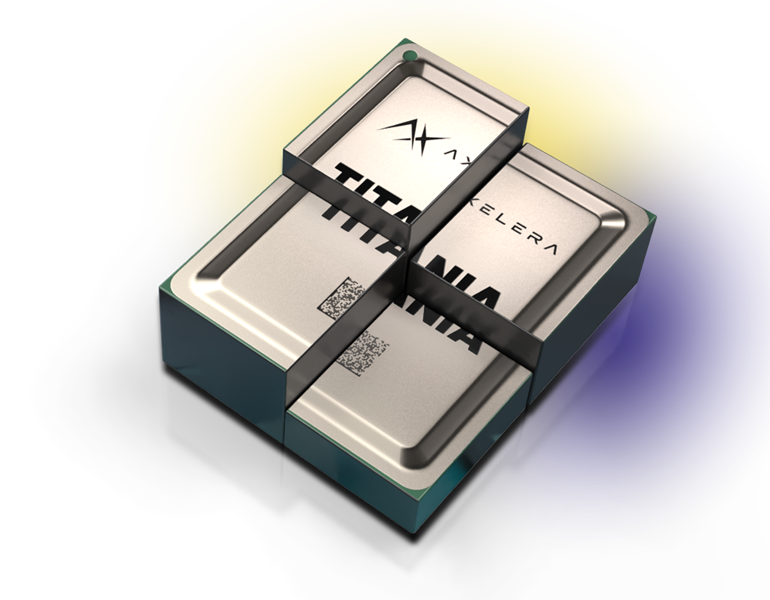
Want to stay up-to-date about our groundbreaking chiplet architecture Titania™?
4. Scaling up and keeping up
Viable scale-up
Once an AI solution for automated inspection has proven effective, the next step involves scaling it up across the manufacturing line or multiple facilities. While the research and development phase involves significant capital expenditure (CAPEX) for model training and optimization, the deployment phase shifts the focus to operational expenditure (OPEX). The key to scaling machine vision for quality control successfully is ensuring that the deployed hardware is both cost-effective and power-efficient, making widespread implementation economically viable.
Axelera AI offers the most cost-effective solution in the market, ensuring that large-scale deployment is economically viable. Our hardware solutions, available in PCIe and M.2 formats, are designed to provide high performance while minimizing power consumption. This balance of efficiency and affordability makes it feasible to deploy advanced AI models across extensive manufacturing operations without escalating operational costs.
Future-proof platform
The field of deep learning is continuously evolving, with new state-of-the-art (SOTA) models and techniques emerging regularly. Innovations such as YOLOv8-Seg, MemoryMamba, EfficientAD or Segment Anything Model (SAM) demonstrate significant advancements in real-time segmentation, memory-augmented state space modeling, advanced and efficient anomaly detection, and zero-shot learning capabilities. For manufacturers, keeping up with these rapid advancements is essential to maintaining a competitive edge and operational efficiency.
Axelera AI is committed to a comprehensive software and chipset roadmap that ensures support for emerging deep learning architectures and technologies such as transformers or LLMs. Our development team stays at the forefront of AI research, integrating breakthroughs quickly into our platform. This ensures that our customers can leverage the latest innovations without delay.
Try out accelerated machine vision in quality control
Axelera AI is at the forefront of AI-driven automated inspection and quality control 4.0, offering robust, adaptable, and cost-effective solutions tailored to the unique challenges of modern manufacturing. With our state-of-the-art hardware acceleration, seamless integration capabilities, and commitment to keeping pace with technological advancements, we ensure your vision inspection systems are optimized for efficiency, accuracy, and innovation.
Do not miss the opportunity to enhance your vision systems for manufacturing. Contact us today to learn more or request a Metis development system. Let Axelera AI be your partner in achieving unparalleled quality and operational excellence.
References
- ScienceDirect. Deep Learning for Defect Detection: Advances and Applications.
- [Springer. A Review of Deep Learning-Based Approaches for Defect Inspection. ]https://link.springer.com/article/10.1007/s12596-023-01340-5)
- IEEE Xplore. “Advances in Deep Learning for Quality Control in Manufacturing.”
- He, K., Zhang, X., Ren, S., & Sun, J. (2016). Deep Residual Learning for Image Recognition. In Proceedings of the IEEE Conference on Computer Vision and Pattern Recognition (CVPR).
- Bochkovskiy, A., Wang, C. Y., & Liao, H. Y. M. (2020). YOLOv4: Optimal Speed and Accuracy of Object Detection. arXiv preprint arXiv:2004.10934.
- Ronneberger, O., Fischer, P., & Brox, T. (2015). U-Net: Convolutional Networks for Biomedical Image Segmentation. arXiv preprint arXiv:1505.04597.
- Kingma, D. P., & Welling, M. (2013). Auto-Encoding Variational Bayes. arXiv preprint arXiv:1312.6114.
- Finn, C., Abbeel, P., & Levine, S. (2017). Model-Agnostic Meta-Learning for Fast Adaptation of Deep Networks. Proceedings of the 34th International Conference on Machine Learning (ICML).
- [Xian, Y., Lampert, C. H., Schiele, B., & Akata, Z. (2018). Zero-Shot Learning - A Comprehensive Evaluation of the Good, the Bad and the Ugly. IEEE Transactions on Pattern Analysis and Machine Intelligence (TPAMI). ]https://arxiv.org/abs/1707.00600)
- Meta AI, 2024. “Segment Anything Model (SAM).” Meta AI
- ArXiv. “MemoryMamba: Memory-Augmented State Space Model for Defect Recognition.”
- MDPI. “Enhanced YOLOv8 with BiFPN-SimAM for Precise Defect Detection in Miniature Capacitors.”
- ArXiv. “EfficientAD: Accurate Visual Anomaly Detection at Millisecond-Level Latencies”